How profitable is embryo selection for IQ in the United States right now?
Profitable, but not that effective.
TL;DR: with current technology, the average couple should be expected to find a gain of at least one IQ point when selecting embryos for only intelligence. This point should translate to about 40,000 to 90,000 dollars in additional lifelong income for the child, which is higher than the cost of engaging in embryo selection.
Theoretically, if a set of embryos are similar in their predispositions to intelligence, other traits such as health or appearance can be selected for instead, meaning that the figure of one point is misleading when it comes to the efficacy of selecting embryos for intelligence.
#######
This post is meant to build on Gwern’s blogpost, much more detailed than this one, though this one includes a more precise estimate of IQ’s effect on lifelong income as well as estimates that are calculated based on current technology.
Estimating the profitability of embryo selection is simple: calculate the cost of selecting the embryos, the effect selecting embryos for intelligence has on IQ, and how much this gain increases lifelong income. The profitability is the gain minus the cost.
The cost of embryo selection
Gwern estimates the cost of IVF to be somewhere between 8,000 and 20,000 USD. This appears to have been accurate at the time, though the cost of IVF appears to have increased in recent years, with sources reporting figures in these ranges: 15,000-30,000, 10,000, 14,000-20,000, 20,000, and 15,000 - 20,000. The absolute interval for the cost is $10,000 - $30,000, and the mean is $18000. (Edit: a friend of mine told me that IVF costs 5k USD in Czechia, and that splitkits can be used to reduce the cost of sequencing the embryos).
Nebula is currently selling whole genome kits for $250, which would be the cost of selecting a single embryo for genes.
There is also an additional cost that arises from choosing to do polygenic testing itself. Gwern finds that, based on public cost data, the price of genetic testing is about $4,000, but that most of this cost is due to the embryo freezing and genetic screening; the former must be done regardless of whether embryo selection is carried out and the cost of the latter can be estimated using kits. The embryo biopsy, which is the necessary process to test embryos genetically costs only about 1,000 - 2,000 USD or so.
This leaves the following formulas to compute the cost of embryo selection:
Total Cost ($) = 18,000 (range of 10,000 - 30,000) + 1,500 (1,000 - 2,000) + 250*Embryos
Marginal Cost ($) = 1,250 (1,000 - 1,500) + 250*Embryos
The expected gain in intelligence
Jonathan Anomaly claims that embryo selection for IQ can result in an average gain of 6 points in the modern day, when the best embryo of 10 is chosen, based on unpublished research. For the purposes of rigour, I will detail why this estimate is defensible.
At the moment, polygenic scores for educational attainment (EA4) correlate at about .30 with measured IQ — the IQ measurement being the Peabody Picture Vocabulary Test (PPVT) in the Add Health dataset. Selecting embryos with only EA4 would lead to a gain of five points if the best of 10 embryos was selected.
Theoretically, selecting embryos based on multiple traits such as type 2 diabetes, ADHD, verbal-numerical reasoning, schizophrenia, or coronary artery disease should lead to larger gains in cognitive ability. Based on modeling done by gwern, selecting embryos based on multiple traits could result in gains 3-5 times larger than those that are found when selection is only based on cognitive ability:
Intelligence is one of the most valuable traits to select on, and one of the easiest to analyze, but we should remember that it is neither necessary nor desirable to select only on a single trait. For example, in cattle embryo selection, selection is done not on a single trait but a weighted sum of 48 traits (Mullaart & Wells 2018).
Re-estimating with higher=better corrected, the original multiple selection turned out to be somewhat overestimated. Adding the real trait heritabilities, we see that the gains to multiple selection remain large compared to single selection (2.9 or 3.3SDs vs 0.6SDs), and that the genetic correlations do not substantially reduce gains to multiple selection but in fact benefits multiple selection by adding +0.35SD.
Personally, I’m a bit skeptical of these numbers. In theory this should work, but the fact that polygenic scores are fit using the same samples makes it unlikely that gains these large would be found in practice. (Edit: I have been told in a PM that this method may work better than I initially suspected).
There is also the issue of noncausal variance in the relationship between polygenic scores and IQ — gwern doesn’t think this is a big issue because polygenic scores are based on passive observation and prediction; as long as some of the causal variants are transmitted, embryo selection will work.
The Prediction Is Noncausal: GWASes may be predictive but this is irrelevant because the SNPs in a PGS are merely non-causal variants which proxy for causal variants
Background: in a GWAS, the measured SNPs may cause the outcome or they may merely be located on a genome nearby a genetic variant which has the causal effect; because genomes are inherited in a ‘chunky’ fashion, a measured SNP may almost always be found alongside the causal genetic variant within a particular population. (Over a long enough timeframe, as organisms reproduce, that part of the genome will be broken up, but this may take centuries or millennia.) Such a SNP is in “linkage disequilibrium” or just LD. Such a scenario is quite common, and may in fact be the case for the overwhelming majority of SNPs in human GWASes. This is both a blessing and a curse for GWASes: it means that easy cheaply-measured SNPs can probe harder-to-find genetic variants, but it also means that the SNPs are not causal themselves. So for example, if one took a list of SNPs from a GWAS, and used CRISPR to edit them, most of the edits would do nothing. This is a serious concern for genetic engineering approaches—just because you have a successful GWAS doesn’t mean you know what to edit!
But is this a problem for embryo selection? No. Because you are not engaged in any editing or causal manipulation. You are passively observing and predicting what is the best embryo in a sample. This does not disturb the LD patterns or break any correlations, and the predictions remain valid. Selection doesn’t care what the causal variants are, it cares only that, whatever they are or wherever they are on the genome, the chosen embryo has more of them than the not-chosen embryos. Any proxy will do, as long as it predicts well. In the long run, changes in LD will gradually reduce the PGS’s predictive power as the SNPs become better/worse proxies, but this is unimportant since there will be many GWASes in between now and then, and one would be upgrading PGSes for other reasons (like their steadily improving predictive power regardless of LD patterns).
Gusev instead has objected to selecting embryos for intelligence (and against the accuracy of genetic predictions of intelligence in general), partly based on this concern. Notably, the direct effect of polygenic scores is lower than the population effect, where the direct effect of the score is estimated by taking the ratio of the effect observed when controlling for parental PGS and the zero-order effect:
By controlling for parental EA PGIs, we isolate the component of predictive power that is due to direct effects, or the causal effects of an individual’s genetic material on that individual. For EA and 22 other phenotypes, controlling for the parental EA PGIs roughly halves the EA PGI’s association with the phenotype. In contrast, when we examine PGIs for height, body mass index (BMI) and cognitive performance, controlling for parental PGIs has far less impact on their associations with their corresponding phenotype.
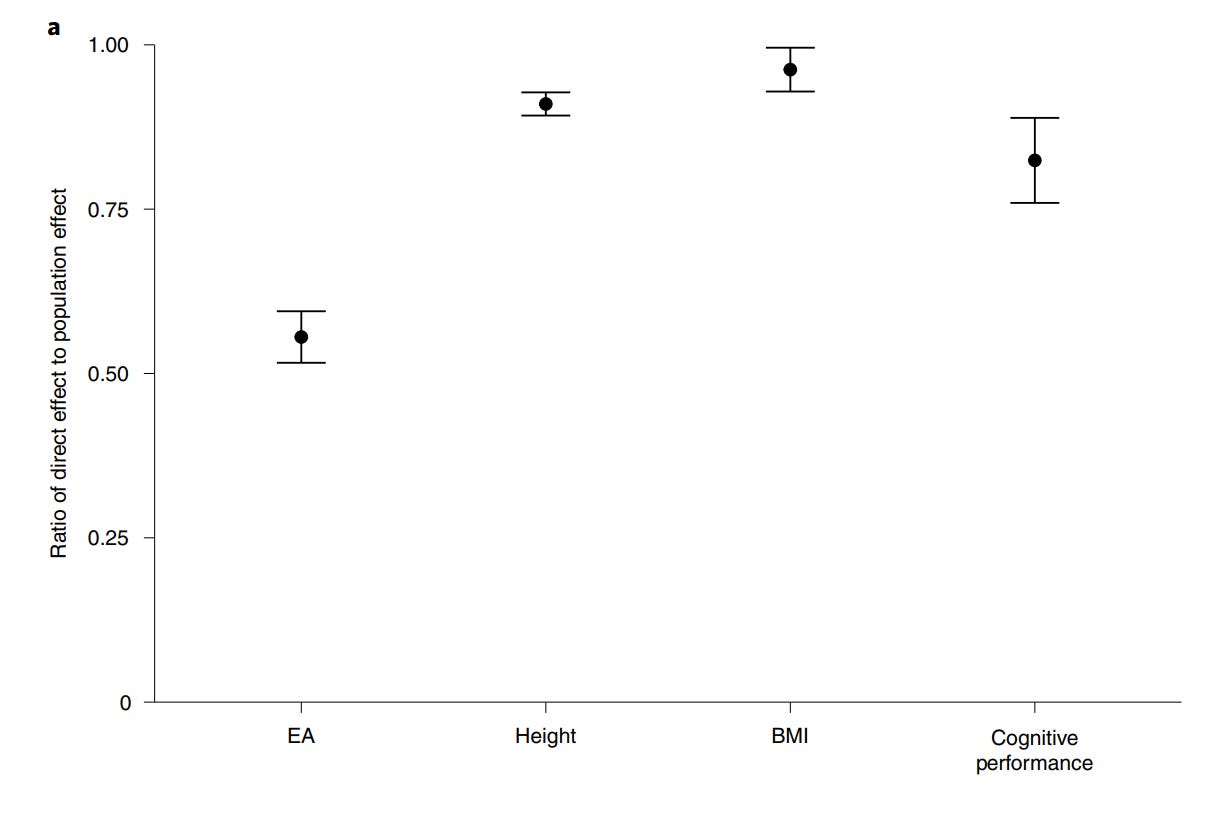
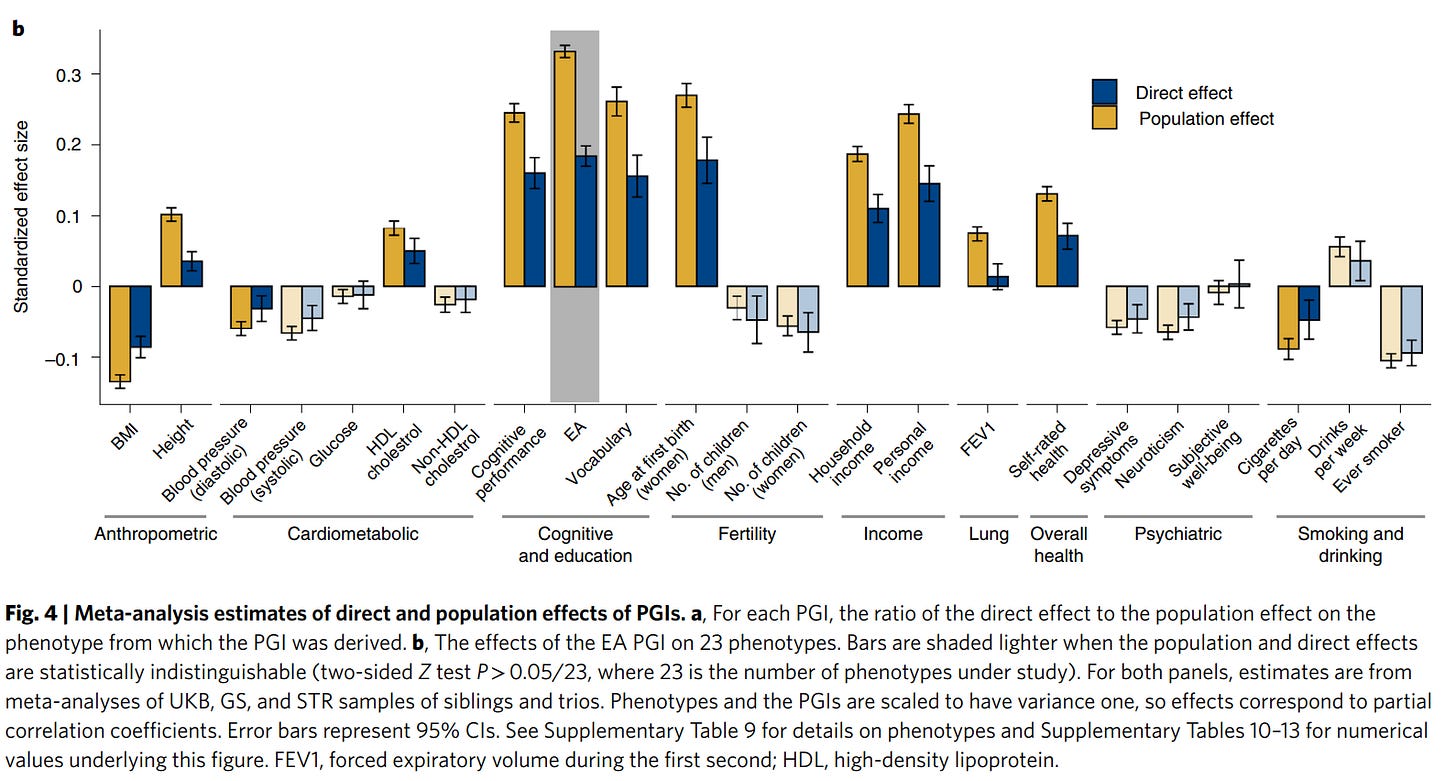
I doubt it is a coincidence that educational attainment, the least heritable of the traits, and the one with the most plausible vertical transmission mechanism is also the one that attenuates the most after controlling for parental polygenic scores. But I find it hard to believe the vertical transmission mechanisms for height and BMI are that large; this result could be a function of regression to the mean, shared causal ancestry, or some other bias that is not known at the moment. It would be prudent see if this method survives scrutiny in the future before making any definitive claims about the existence of vertical transmission effects or noncausal variance. Despite these concerns, the ratio of the direct effect to the population effect is still high for cognitive performance (~.75), so the issue of noncausal variance is not that relevant for the prediction of intelligence itself.
Besides this issue, Gusev notes that the predictive validity of polygenic scores is lower in non-White populations. This is correct, but this is not much of a concern, as the majority of the people using this technology will likely be White.
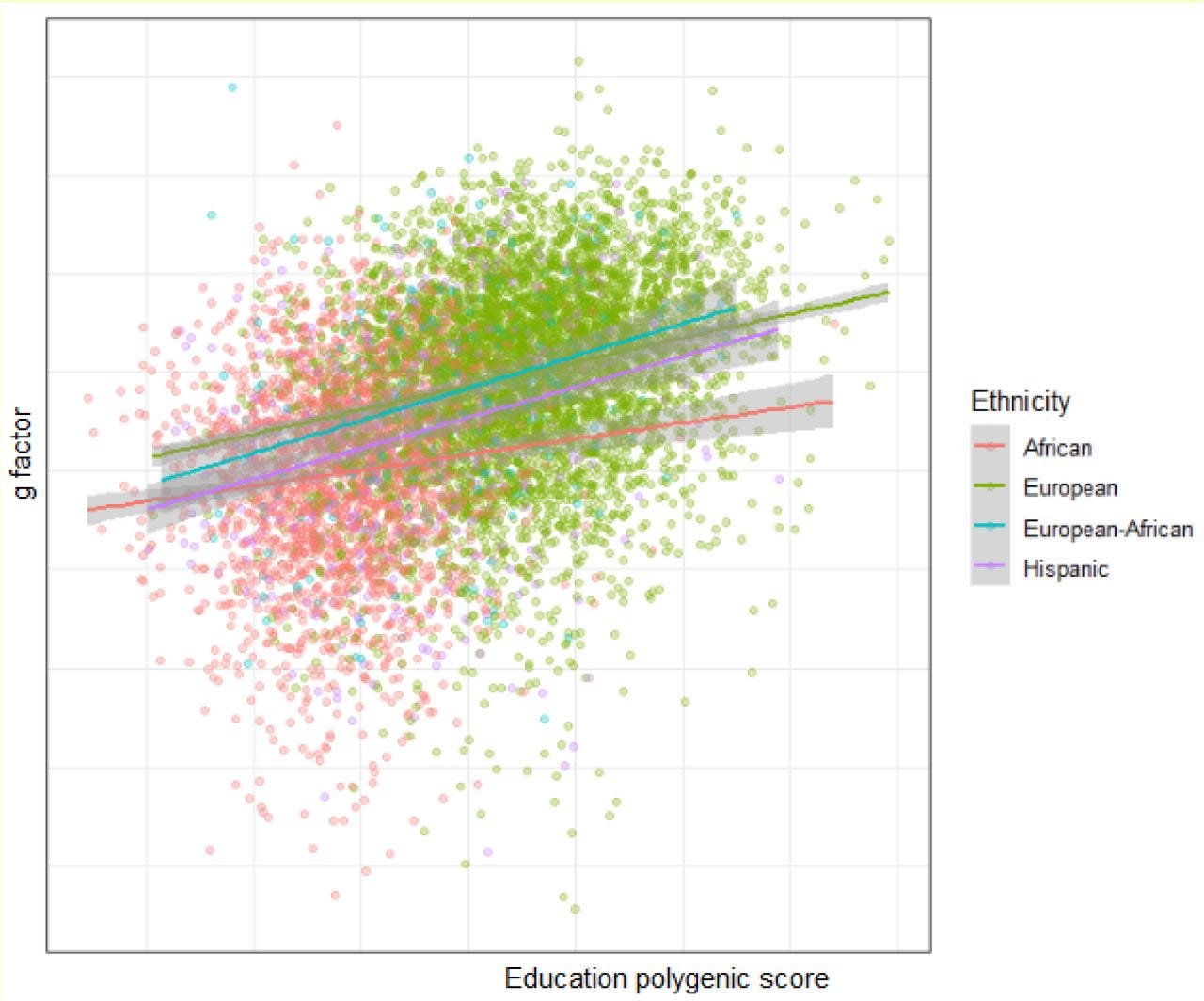
Based on a table I created using simulations, Anomaly’s estimate of an average gain of 6 points would imply an causal correlation between PGS and IQ of roughly .37. It turns out this guess was accurate, and based on a Based on the evidence available, there is no reason to doubt this statement.
II.
Unfortunately, the scenario of a woman giving 10 eggs and being able to choose the best is an optimistic scenario. Women bear varying numbers of eggs, some of the implantations will inevitably fail, and some of the pregnancies will end in miscarriages. Fortunately, gwern has already constructed a model to account for these events:
In vitro fertilization is a sequential probabilistic process:
harvest x eggs
fertilize them and create x embryos
culture the embryos to either cleavage (2–4 days) or blastocyst (5–6 days) stage; of them, y will still be alive & not grossly abnormal
freeze the embryos
optional: embryo selection using quality and PGS
unfreeze & implant 1 embryo; if no embryos left, return to #1 or give up
if no live birth, go to #6
Using figures derived from studies of two IVF populations (now called the Tan and Hodes-Wertz samples), gwern models gains of 0.38 IQ points (Tan sample) and 0.68 points (Hodes-Wertz sample), assuming the embryos are selected based on a previous GWAS from 2016. Using the most recent estimate (r = .37 between PGS and IQ), these figures increase to .79 and 1.35 respectively.
It is worth mentioning that these estimates likely underestimate the true effect within the general population, as the samples these figures are based on appear to be selected for fertility troubles. Gwern claims that harvesting 10-30 eggs is common; I’ve also been told this by people who have done IVF.
The Hodes-Wertz study reported on outcomes of 287 cycles of IVF with 24-chromosome PGS with a total of 2,282 embryos followed by fresh day-5 embryo transfer in RPL patients. Of the PGS cycles, 67% were biopsied on day 3, and 33% were biopsied on day 5. The average maternal age was 36.7 years (range: 21-45 years), and the mean number of prior miscarriages was 3.3 (range: 2-7)
The Tan sample:
A total of 395 couples participated. They were carriers of either translocation or inversion mutations, or were patients with recurrent miscarriage and/or advanced maternal age. A total of 1,512 blastocysts were biopsied on D5 after fertilization, with 1,058 blastocysts set aside for SNP array testing and 454 blastocysts for NGS testing. In the NGS cycles group, the implantation, clinical pregnancy and miscarriage rates were 52.6% (60/114), 61.3% (49/80) and 14.3% (7/49)
IQ and Income
Most research on the subject agrees that IQ and income are correlated, and that the causality goes from IQ to income. The largest meta-analysis on this subject finds the mean correlation between IQ and income is .21, but looking through the studies they cited, I find several issues:
Low quality testing: imperfect measures such as vocabulary tests or raven’s tests are used as proxies for g, which predictably result in lower correlations.
Non-representative samples: for example, the WLS is a sample of high school graduates from Wisconsin, where the correlation is predictably smaller, as part of the reason IQ correlates with income is because of educational attainment.
Some correlations were measured in young adults, which tend to be lower.
Nationality: the relationship between IQ and income is unusually large in America.
To estimate the effect within the United States, I consider the NLSY to be the best dataset, since it is a large (n > 10,000 between the two waves), representative sample that tests IQ well (ASVAB), and has income data derived from multiple years. Here, I present the uncontrolled effect of one IQ point on yearly income by age and cohort:
The raw effect of IQ on income increases as individuals age, largely because the variance in income increases (details in Appendix). Using these summary statistics, the effect of an increase in one IQ point has on lifelong income was estimated by simply regressing the raw effect onto age. This regression model estimated that one IQ point increases total income from the ages of 25 to 65 by $50,586.
#attempt contains the summary statistics, raweffect is the effect of one IQ point on income in dollars, and age is the expected average age of the respondents at this year.
#only ages 25 to 65 are predicted because the income years have restricted ages.
reg <- lm(data=attempt, raweffect ~ age)
preds <- predict.lm(reg, newdata=data.frame(age=seq(from=25, to=65, by=1)))
sum(preds)
[1] 50586.33
Assuming the effect of one IQ point on income is 100$ in people between the ages of 18 and 24 (it’s $133 at 24), and 1000$ between the ages of 66 and 80 (it’s $1,986 at 60), the true effect of one IQ point on lifelong income is about $66,286.
If the estimate were only based on the more recent cohort (NLSY97), the estimated effect between the ages of 25 and 65 would be $74,508, and adding the rough estimates for the additional years (18-24 and 66-80) increases it to $90,208. I think this estimate is too high; this projection relies on the assumption that the increases in the mean and variance of wages will hold for the future, which may not be the case.
reg <- lm(data=attempt %>% filter(dataset=='NLSY97'), raweffect ~ age)
preds <- predict.lm(reg, newdata=data.frame(age=seq(from=25, to=65, by=1)))
sum(preds)
[1] 74508.14
This calculation has both upwards biases (confounding with region, race, and family background) and downwards biases (the slope is larger in recent years, measurement error in intelligence, and measurement error in income due to self-reporting), which leads me to believe that the estimate itself is unbiased but likely inaccurate.
For now, I’ve pegged the upper and lower limits at $90,000 and $40,000 respectively.
The profitability
Based on the statistics I have estimated earlier, the marginal and total profitability can be calculated.
The lowest expected gain due to embryo selection is $31,600 (.79 x $40,000) and the highest is $121,500 ($90,000 x 1.35), the lowest expected marginal cost is $1,600 ($1,000 + $200 x 3) and the highest is $3,640 ($2000 + $200 x 8.2), the lowest expected total cost is $11,650 (10000 + 1,000 + 200 x 3), and the highest expected total cost is $33,640 (30000+2000 + 200 x 8.2). It appears that, regardless of what type of cost is calculated or what parameters are chosen, embryo selection is profitable.
This statistic is misleading because the profitability of embryo selection will depend massively on the individual and the situation. Would embryo selection work on a British 22 year old girl who had 34 eggs harvested from her? Most definitely. The 40 year old Chinese woman who could only muster 3 eggs? I wouldn’t bet on it. If bad luck strikes, and all embryos are about equal in their predispositions to intelligence, other phenotypes such as health or appearance can be selected for instead.
Appendix
Code replication of gwern’s finding that a polygenic score that explains 33% of the variance in IQ will lead to an expected gain of 9.42 points if the best of 10 embryos are selected:
rookie <- rnorm(100000, 0, 0.71)
rk <- length(rookie)/10
maxim = rep(0, rk)
for(i in 1:rk) {
mind = 1 + (i-1)*10
maxd = i*10
maxi <- max(rookie[mind:maxd])
maxim[i] <- maxi
}
mean(maxim)*sqrt(0.33)*15
[1] 9.41383
Code for embryo models (taken from gwern):
currentpgs <- 0.37^2
selzam2016 <-0.035
simulateIVF <- function (eggMean, eggSD, polygenicScoreVariance, normalityP=0.5, vitrificationP, liveBirth) {
eggsExtracted <- max(0, round(rnorm(n=1, mean=eggMean, sd=eggSD)))
normal <- rbinom(1, eggsExtracted, prob=normalityP)
scores <- rnorm(n=normal, mean=0, sd=sqrt(polygenicScoreVariance*0.5))
survived <- Filter(function(x){rbinom(1, 1, prob=vitrificationP)}, scores)
selection <- sort(survived, decreasing=TRUE)
if (length(selection)>0) {
for (embryo in 1:length(selection)) {
if (rbinom(1, 1, prob=liveBirth) == 1) {
live <- selection[embryo]
return(live)
}
}
}
}
simulateIVFs <- function(eggMean, eggSD, polygenicScoreVariance, normalityP, vitrificationP, liveBirth, iters=100000) {
return(unlist(replicate(iters, simulateIVF(eggMean, eggSD, polygenicScoreVariance, normalityP, vitrificationP, liveBirth))));
}
simulateTan <- function() {
return(simulateIVFs(3, 4.6, currentpgs, 0.5, 0.96, 0.24))
}
iqTan <- mean(simulateTan()) * 15; iqTan
iqTan
0.7509493
simulateHodesWertz <- function() {
return(simulateIVFs(8.2, 4.6, currentpgs, 0.35, 0.96, 0.40)) }
iqHW <- mean(simulateHodesWertz()) * 15
iqHW
1.349667
I was also able to replicate his results that used the h^2 = .035 estimate from Selzam:
simulateTan <- function() {
return(simulateIVFs(3, 4.6, selzam2016, 0.5, 0.96, 0.24))
}
iqTan <- mean(simulateTan()) * 15; iqTan
0.3805512
simulateHodesWertz <- function() {
return(simulateIVFs(8.2, 4.6, selzam2016, 0.35, 0.96, 0.40)) }
iqHW <- mean(simulateHodesWertz()) * 15
iqHW
0.6871452
Here I present two tables, which contain various parameters of interest from the NLSY datasets:
year: the year at which income was measured.
r: the correlation between IQ and income.
raweffect: the effect an increase of one IQ point has on income.
expeffect: the effect an increase of one IQ point has on % change in income.
sdincome: the standard deviation of income.
These are the results for both datasets:
NLSY79 (born in 1957-1963).
NLSY97 (born in early 80s).
Anomalous results (outlier income data in 1989 and 1992 not removed from the NLSY79)
Code for effect of one IQ point on income between 22 and 65:
> reg <- lm(data=attempt, raweffect ~ age)
> summary(reg)
Call:
lm(formula = raweffect ~ age, data = attempt)
Residuals:
Min 1Q Median 3Q Max
-169.99 -119.92 -45.56 75.54 457.53
Coefficients:
Estimate Std. Error t value Pr(>|t|)
(Intercept) -957.358 105.351 -9.087 5.53e-10 ***
age 48.693 2.682 18.155 < 2e-16 ***
---
Signif. codes: 0 ‘***’ 0.001 ‘**’ 0.01 ‘*’ 0.05 ‘.’ 0.1 ‘ ’ 1
Residual standard error: 153.9 on 29 degrees of freedom
Multiple R-squared: 0.9191, Adjusted R-squared: 0.9163
F-statistic: 329.6 on 1 and 29 DF, p-value: < 2.2e-16
> preds <- predict.lm(lr, newdata=data.frame(age=seq(from=22, to=65, by=1)))
> sum(preds)
[1] 51074.05
Loved the article, just a point on the finance side: as others noted, you should apply a discount rate. If I pay 10,000 USD today and receive 10,000 USD back in 20 years, I did not breakeven, I lost money. This has to be accounted for.
Great article, but one minor nitpick:
“as the majority of the people using this technology will likely be White.”
This would be true initially, but perhaps not eventually, and certainly not worldwide long-term.