Few, if any, human traits correspond to a true normal distribution.
The mean height of men globally is about 171cm, with a standard deviation of 7.6 cm. If normality held, it would be close to impossible for there to be people with heights 6.2 standard deviations above the mean, yet there are plenty of people who are taller than 218cm (7’2’’). Even if the average global height were equal to that of European-descended men (180cm), the maximum height according to the normal distribution would be 227cm (7’6’’). This is empirically not supported either — there are five people who are 244cm or taller who live today.
Disorders like gigantism cause people to grow to heights that are beyond what is expected from the sum of regular additive genetic factors and environments, and the same holds for dwarfism, which combine cause height to have a leptokurtic distribution: one with many outliers.
Another violation of normality that is seen in statistical distributons is skewness, where the tail of the distribution is skewed towards one side of the distribution. In the case of BMI, the tail is more prominent at the right side of the distribution, while for age at death the opposite is the case. This is probably due to the fact that there are more ways to shift to one side of the distribution than the other: there are many ways to die, but only one to live — not die.
Violations of normality can also arise from multiplicative distributions. If a trait is influenced by many additive genetic and environmental effects, the distribution will be close to normal, while the distribution of a trait that is influenced by many multiplicative effects will follow a log-normal distribution, which is equivalent to a normal distribution that has been log-transformed. In 97-99% of the population, income follows a log-transformed distribution.
As humans have been selected for intelligence for at least 10,000 years, genetic variants that have strong effects on intelligence will have largely reached fixation within humans. This means that when mutations cause these alleles to change, they will usually result in reductions to intelligence. Empirically, rare variants associated with education overwhelmingly tend to have large negative effects on the trait.
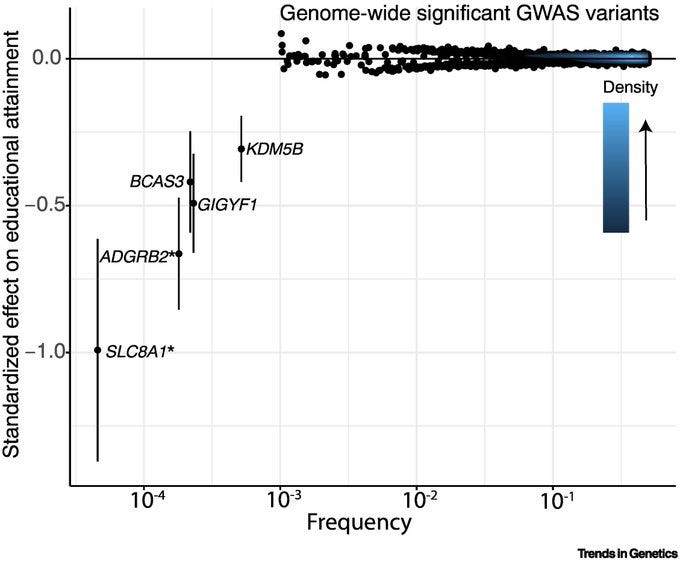
If this is the case, then mutations should shift the distribution of intelligence towards the left, similar to the way dwarfism causes lots of outliers in terms of height.
The best dataset to test this theory out is the Project Talent, a study of American highschoolers, due to the fact that it has a many subtests (59, including the iffy ones) and tested a large number of individuals (~370,000). People that were either under 14 or over 18 were removed to avoid selection biasing the age effects, and the sample was subset to White, non-Jewish men with data on their ages. This left 68,000 individuals, which is more than enough to test for the existence of normality.
To calculate levels of intelligence, the first unrotated principal component was extracted out of the 59 tests, with age partialled out afterwards. The distribution of scores is as follows:
The scores had a skew and kurtosis of -0.26, indicating that the distribution was a left-tailed distribution with a relative lack of outliers. The standard errors of these estimates are extremely low: 0.01 for skewness and 0.02 for kurtosis, so the estimates would pass any conventional significance testing.
The minimum score was 38.4, while the maximum score was 141.1. If these scores followed a normal distribution, it would be expected that the top score would be about 160, a score 50% more extreme than the one that was actually observed.
A nice vindication of the theory, but it would be prudent to test whether this an artefact of violations in normality observed within the individual subtests. To do this, the subtests were transformed to a rank-ordered distribution which was then converted to a normal distribution. That caused the subtests to look more normal, but all of them had a kurtosis of ~0.2 and skewness of ~-0.2. To fix that, a Yeo-Johnson transformation was applied, though many subtests were still not normally distributed. The van der Waerden transformation was applied to this transformed data after the first transformation, and now 13 subtests finally had a skewness and kurtosis of between -0.1 and 0.1. A general score was calculated from these tests, age was controlled for, and finally, the distribution was plotted.
Roughly the same distribution, but with less kurtosis.
There is no guarantee that this finding applies outside of this dataset, so I checked three other datasets that had more than 9 subtests of intelligence. Even after the subtests were deliberately manipulated to fit a normal distribution, as was done before, in all 3 cases, the factor scores had a skewness of between -0.2 and -0.3.
If the individual subtests cannot be blamed for the artefact, then that implies that the true distribution of intelligence is indeed left-tailed and possibly deficient in outliers as well. Which is interesting — this supports the theory that Spearman’s Law of Diminishing Returns is an artefact of intelligence not having a normal distribution. This does not necessarily imply that general intelligence has the same effect on cognitive performance at each part of the distribution, just that Spearman’s Law cannot be cited to state this is the case.
Edit — Inquisitive bird mentions Johnson et al (2008) who also find evidence for a non-normal distribution within the Scottish Mental Surveys of 1932 and 1947, both of which tested most of Scotland’s 11 year olds:
The most obvious departure was that neither distribution was symmetric: in each survey, more scores were below the peak of the distribution than above it, indicating negative skewness. Skewness was -.211 and -.347 in SMS32 and SMS47, respectively. These skewness values do not sound excessive by common standards, but they were highly statistically significant in these large samples. If we want to understand the distribution of general intelligence at the level of the population, these skewness results matter.
Edit 2 — there were a few goofs in the original post: I stated there were 61 subtests instead of 59 and that the average height of Europeans was 180cm.
Edit 3 — mostly wording.
Always great to see this discussed. IQ is less like height and more like mountains - rarefied tall outliers skew the average height up, obscuring the many minute foothills. Similar writings by Nassim Taleb, and which align with my own experience, suggests a skew even more extreme than presented here.
The left tail theory is supported by the iq distributions of siblings and co-twins when one has mild vs severe ID(the large swedish and israeli studies were posted by cremieux on twitter). It's just the case that most severe ID(and to some extent mild) cases are the result of a developmental issue in a gentile white american population. And the frequency is roughly the same in populations, so I wonder if differences in the standard deviation can be explained by that. But I'm not sure this explains SLODR, if anything mentally disabled individuals have a lower g loading/measurement non-invariance(https://www.research.unipd.it/bitstream/11577/3196682/1/Giofr%C3%A8%2C%20D.%2C%20%26%20Cornoldi%2C%20C.%20(2015).%20The%20structure%20of%20intelligence%20in%20children%20with%20specific%20learning%20disabilities%20is%20different%20as%20compared%20to%20typically%20development%20children.%20Intelligen.pdf). Height has roughly the same distribution as intelligence with a left tail, and similarly rare cases of gigantism/savants have minimal impact on the distribution.